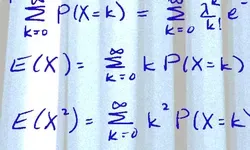
Statistical Inference and Hypothesis Testing in Data Science Applications





This course will teach students about the theory and implementation of hypothesis testing in data science applications. Students will learn to use hypothesis tests to make informed decisions from data, including the general logic of hypothesis testing, error and error rates, power, simulation, and the correct computation and interpretation of p-values. The ethical implications of misuse of testing concepts will also be discussed. This course is part of the MS-DS degree offered on the Coursera platform.▼
Course Feature
Cost:
Free
Provider:
Coursera
Certificate:
Paid Certification
Language:
English
Start Date:
22nd May, 2023
Course Overview
❗The content presented here is sourced directly from Coursera platform. For comprehensive course details, including enrollment information, simply click on the 'Go to class' link on our website.
Updated in [May 25th, 2023]
What skills and knowledge will you acquire during this course?
Students will acquire skills and knowledge in statistical inference and hypothesis testing in data science applications. They will learn the theory and implementation of hypothesis testing, with a focus on its application in data science. The course will cover topics such as the general logic of hypothesis testing, error and error rates, power, simulation, and the computation and interpretation of p-values. Special attention will be given to the misuse of testing concepts, particularly p-values, and the ethical implications of such misuse. This course is part of CU Boulder's Master of Science in Data Science (MS-DS) degree program, which is an interdisciplinary program that brings together faculty from various departments.
How does this course contribute to professional growth?
The course "Statistical Inference and Hypothesis Testing in Data Science Applications" contributes to professional growth by providing students with the knowledge and skills necessary to effectively use hypothesis tests in data analysis. By understanding the general logic of hypothesis testing, error rates, power, and the correct computation and interpretation of p-values, students will be able to make informed decisions based on data. Additionally, the course addresses the misuse of testing concepts, particularly p-values, and highlights the ethical implications of such misuse. This course is part of CU Boulder's Master of Science in Data Science (MS-DS) degree program, which is an interdisciplinary program that brings together faculty from various departments. The MS-DS program is designed for individuals with diverse educational backgrounds and professional experience in computer science, information science, mathematics, and statistics.
Is this course suitable for preparing further education?
This course on Statistical Inference and Hypothesis Testing in Data Science Applications is suitable for preparing further education. It focuses on theory and implementation of hypothesis testing, specifically in the context of data science applications. Students will learn how to use hypothesis tests to make informed decisions based on data. The course covers topics such as the general logic of hypothesis testing, error rates, power, simulation, and the correct computation and interpretation of p-values. It also addresses the misuse of testing concepts, particularly p-values, and the ethical implications of such misuse. This course is part of CU Boulder's Master of Science in Data Science (MS-DS) degree program, which is offered on the Coursera platform. The MS-DS degree is interdisciplinary, involving faculty from various departments including Applied Mathematics, Computer Science, and Information Science.
Pros & Cons
-
Thoughtful and wellstructured course
-
Engaging instructor
-
Indepth material
-
Convincing and wellexplained content
-
Great teachers
-
Excellent applications
-
Unfinished course
-
Unreliable autograder system
-
Not enough student work
-
Limited reference material
-
Complicated Coursera UI
Course Provider
