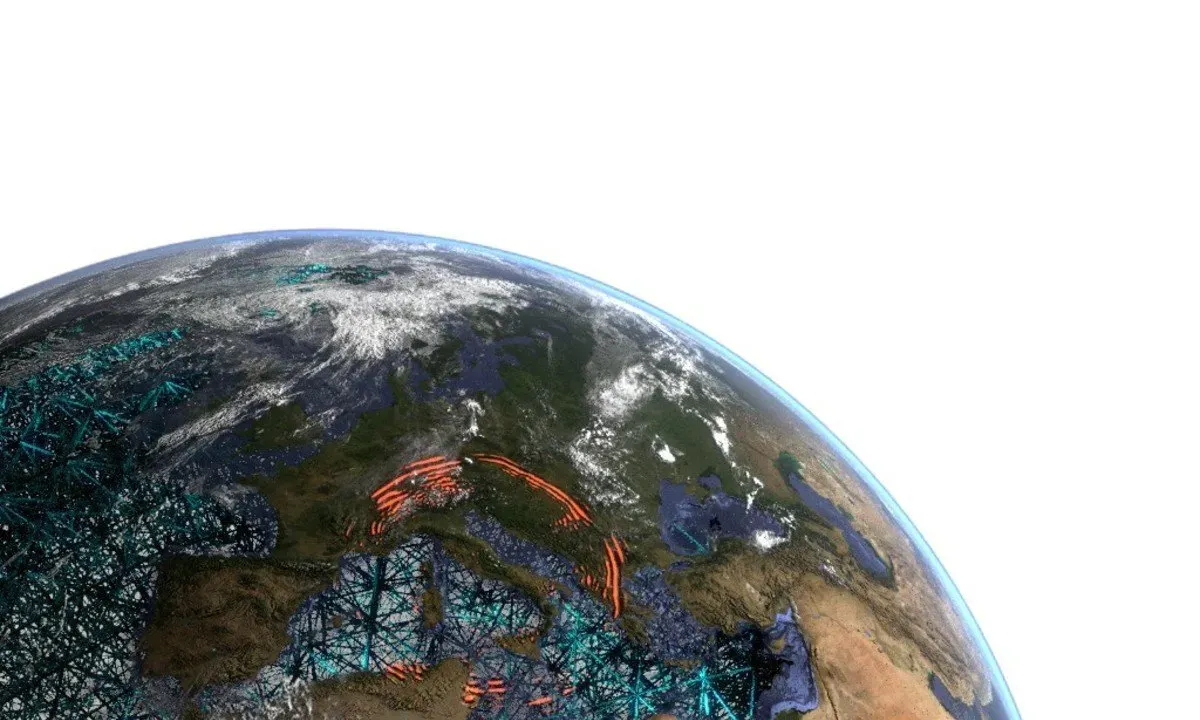
Computers Waves Simulations: A Practical Introduction to Numerical Methods using Python





This course provides a practical introduction to numerical methods for solving partial differential equations, with an emphasis on transforming mathematical equations into python codes and visualizing the results. It covers topics such as Taylor series, Fourier series, differentiation, function interpolation, numerical integration, wave physics, discretization, meshes, parallel programming, and computing models. Strategies for ensuring correct solutions are also discussed.▼
Course Feature
Cost:
Free
Provider:
Coursera
Certificate:
Paid Certification
Language:
English
Start Date:
17th Jul, 2023
Course Overview
❗The content presented here is sourced directly from Coursera platform. For comprehensive course details, including enrollment information, simply click on the 'Go to class' link on our website.
Updated in [March 06th, 2023]
Course Overview:
This course provides a practical introduction to numerical methods for solving partial differential equations using Python. It covers the mathematical derivation of computational algorithms, strategies for ensuring solutions are correct, and basic introductions to wave physics, discretization, meshes, parallel programming, and computing models. It also covers the finite-difference method, the pseudospectral method, the linear and spectral element method, Taylor series, Fourier series, differentiation, function interpolation, and numerical integration.
Possible Development Directions:
This course provides a basic introduction to numerical methods for solving partial differential equations. It is suitable for anyone who is interested in developing or using numerical methods applied to partial differential equations. It can be used as a starting point for further development in natural sciences, engineering, economics, and other fields.
Related Learning Suggestions:
To get the most out of this course, it is recommended to have a basic understanding of Python and partial differential equations. It is also recommended to have a basic understanding of wave physics, discretization, meshes, parallel programming, and computing models. Additionally, it is beneficial to have a basic understanding of Taylor series, Fourier series, differentiation, function interpolation, and numerical integration.
[Applications]
The application of this course is to provide a practical introduction to numerical methods using Python for solving partial differential equations. After completing this course, participants will have a better understanding of the mathematical ingredients of various numerical methods, such as Taylor series, Fourier series, differentiation, function interpolation, and numerical integration. They will also be able to develop and use numerical methods to solve partial differential equations, as well as understand the basics of wave physics, discretization, meshes, parallel programming, and computing models. Furthermore, participants will be able to ensure their solutions are correct by using strategies such as benchmarking with analytical solutions or convergence tests.
[Career Paths]
1. Computational Scientist: Computational scientists use numerical methods to solve complex problems in a variety of fields, such as physics, engineering, and economics. They develop and implement algorithms to solve problems, analyze data, and create simulations. They also use high-performance computing to optimize the performance of their algorithms. This field is rapidly growing, as more and more organizations are turning to computational scientists to solve their most complex problems.
2. Data Scientist: Data scientists use a variety of techniques to analyze large datasets and uncover patterns and insights. They use machine learning algorithms to build predictive models, and use data visualization to communicate their findings. Data scientists are in high demand, as organizations are increasingly relying on data-driven decision making.
3. Software Engineer: Software engineers use programming languages to develop software applications. They design, develop, and test software applications, and use numerical methods to optimize the performance of their applications. Software engineering is a rapidly growing field, as more and more organizations are turning to software engineers to develop their applications.
4. Machine Learning Engineer: Machine learning engineers use machine learning algorithms to build predictive models. They use numerical methods to optimize the performance of their models, and use data visualization to communicate their findings. Machine learning engineers are in high demand, as organizations are increasingly relying on machine learning to make decisions.
[Education Paths]
1. Bachelor of Science in Computer Science: This degree path provides students with a comprehensive understanding of computer science fundamentals, including programming, software engineering, computer architecture, and algorithms. Students will also learn about the latest developments in computer science, such as artificial intelligence, machine learning, and data science. This degree path is ideal for those interested in developing and applying numerical methods to partial differential equations.
2. Master of Science in Applied Mathematics: This degree path provides students with a deep understanding of mathematical principles and their application to real-world problems. Students will learn about numerical methods, optimization, and probability and statistics, as well as their application to partial differential equations. This degree path is ideal for those interested in developing and applying numerical methods to partial differential equations.
3. Doctor of Philosophy in Computational Science: This degree path provides students with a comprehensive understanding of computational science, including numerical methods, computer simulation, and data analysis. Students will learn about the latest developments in computational science, such as artificial intelligence, machine learning, and data science. This degree path is ideal for those interested in developing and applying numerical methods to partial differential equations.
4. Master of Science in Data Science: This degree path provides students with a comprehensive understanding of data science fundamentals, including programming, software engineering, machine learning, and data analysis. Students will also learn about the latest developments in data science, such as artificial intelligence, natural language processing, and deep learning. This degree path is ideal for those interested in developing and applying numerical methods to partial differential equations.
Pros & Cons
-
Clear explanation of concepts
-
Good foundation for more advanced study
-
Excellent supplementary materials
-
Detailed codes and explanations
-
Well thought quizzes system
-
Complications quickly arise
-
Not mathematically derived
-
No followup course
-
No advanced material covered
Course Provider
